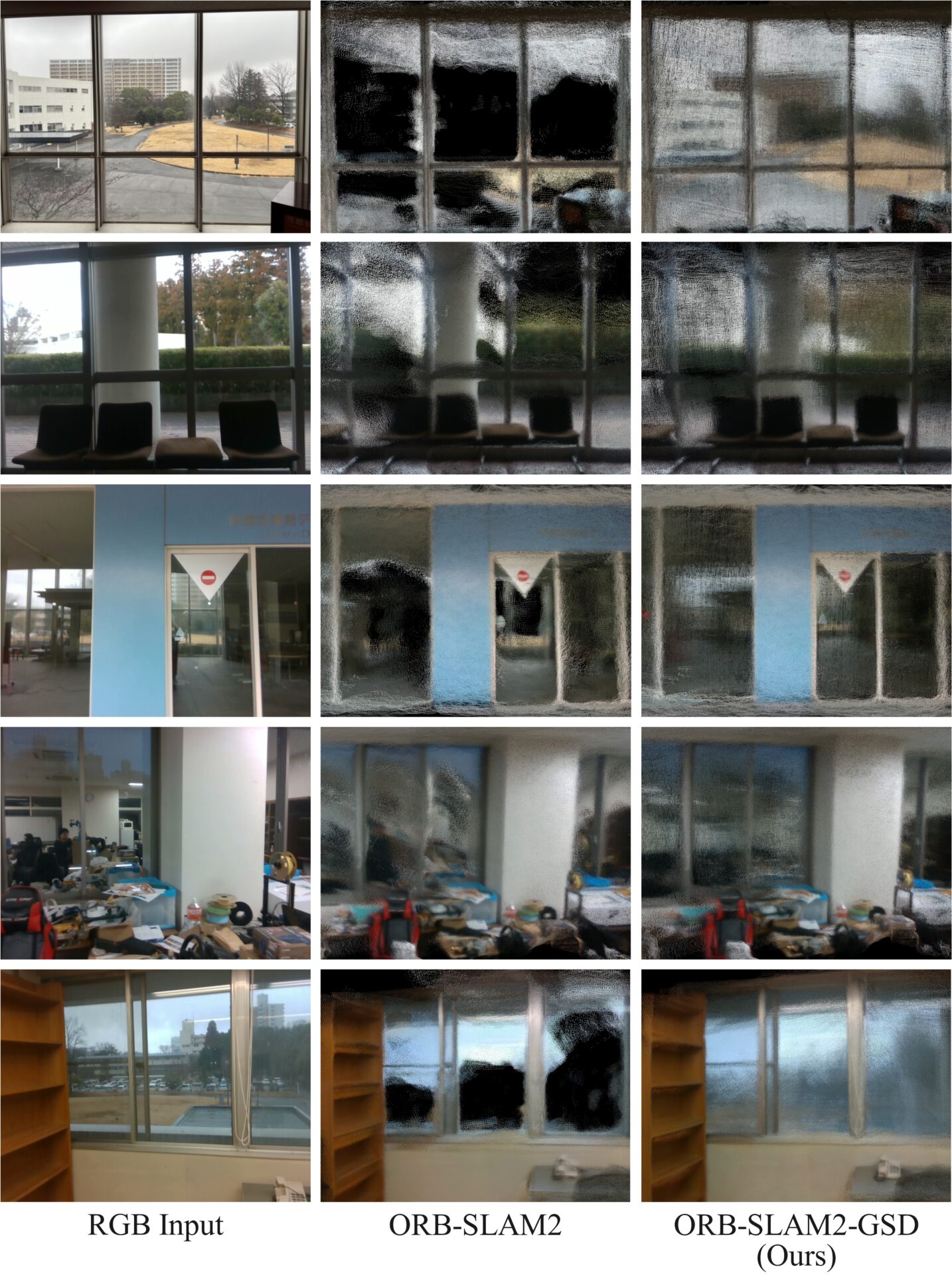
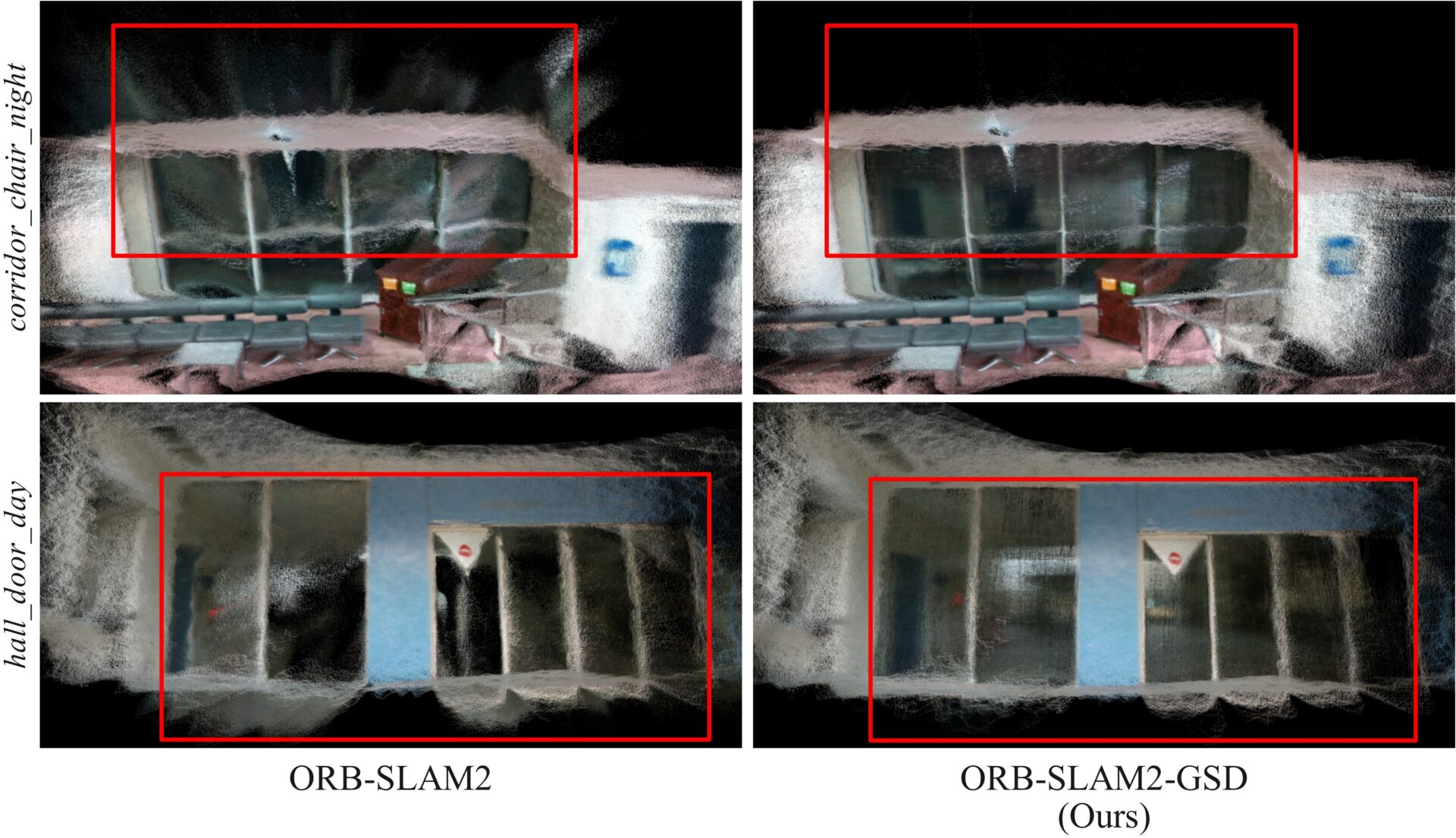
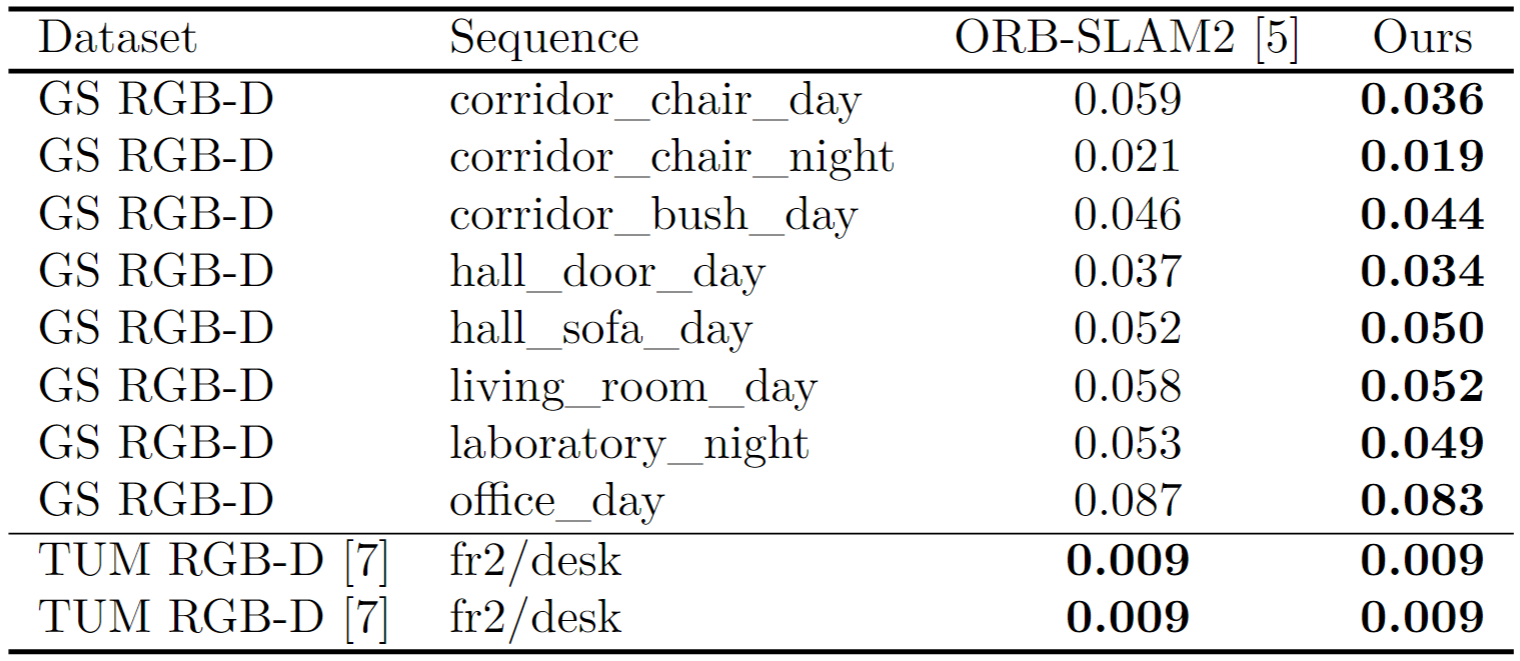
The widespread presence of glass surfaces such as glass windows, glass walls, and glass doors poses challenges to the use of RGB-D SLAMs in modern indoor scenes. The performance of RGB-D SLAMs is highly dependent on the accuracy of the depth maps obtained. If the depth values of objects are significantly erroneous, it introduces substantial noise into the mapping and camera trajectory estimation processes. Unfortunately, whether using time of flight (ToF) systems or stereo camera systems, depth cameras are unable to accurately capture the depth values of glass surfaces or the objects behind them, as illustrated in the red dotted box in the following figure.
ガラス窓、ガラス壁、ガラスドアなどのガラス表面が広く存在することにより、現代の室内環境におけるRGB-D SLAMの利用には問題が生じている。RGB-D SLAMの性能は、取得される深度マップの精度に大きく依存する。物体の深度値が大幅に誤っている場合、マッピングやカメラの軌跡推定に重大なノイズが発生する。残念ながら、ToF(Time of Flight)システムやステレオカメラシステムを使用する場合でも、深度カメラはガラス表面やその背後にある物体の深度値を正確に捉えることができない。以下の図の赤い点線の枠内で示されている。
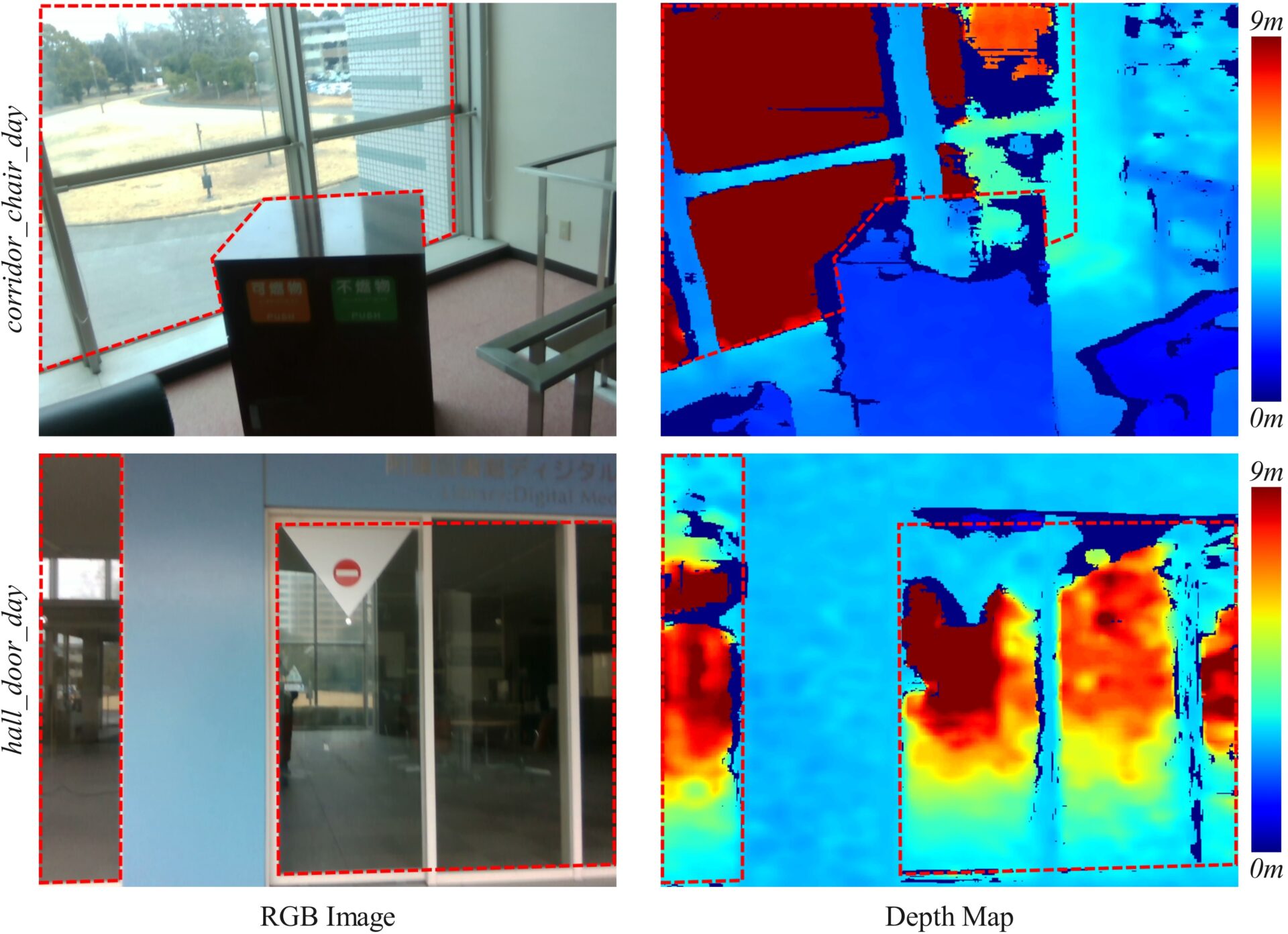
The above reasons make it difficult for RGB-D SLAMs like ORB-SLAM2 to ensure the accuracy of depth measurements for feature points in indoor environments with many glass surfaces. This leads to inaccuracies in the positions of the constructed map points. Incorrect map points directly affect the estimation of the camera pose, causing a decline in the accuracy of camera trajectory estimation. In turn, imprecise camera poses further degrade the accuracy of subsequent map point construction. This vicious cycle results in suboptimal camera trajectory estimation and mapping performance for RGB-D SLAMs in glass surface scenes, as shown in the following figure.
以上の理由から、多くのガラス表面が存在する室内環境では、ORB-SLAM2のようなRGB-D SLAMが特徴点の深度測定の精度を確保することが困難になる。その結果、構築されたマップポイントの位置に誤差が生じる。誤ったマップポイントはカメラの姿勢推定に直接影響を与え、カメラ軌道推定の精度が低下する。不正確なカメラの姿勢は、後続のマップポイント構築の精度をさらに悪化させる。この悪循環により、ガラス表面シーンにおいてRGB-D SLAMのカメラ軌跡推定およびマッピング性能が良くない、以下の図に示されるような結果となる。
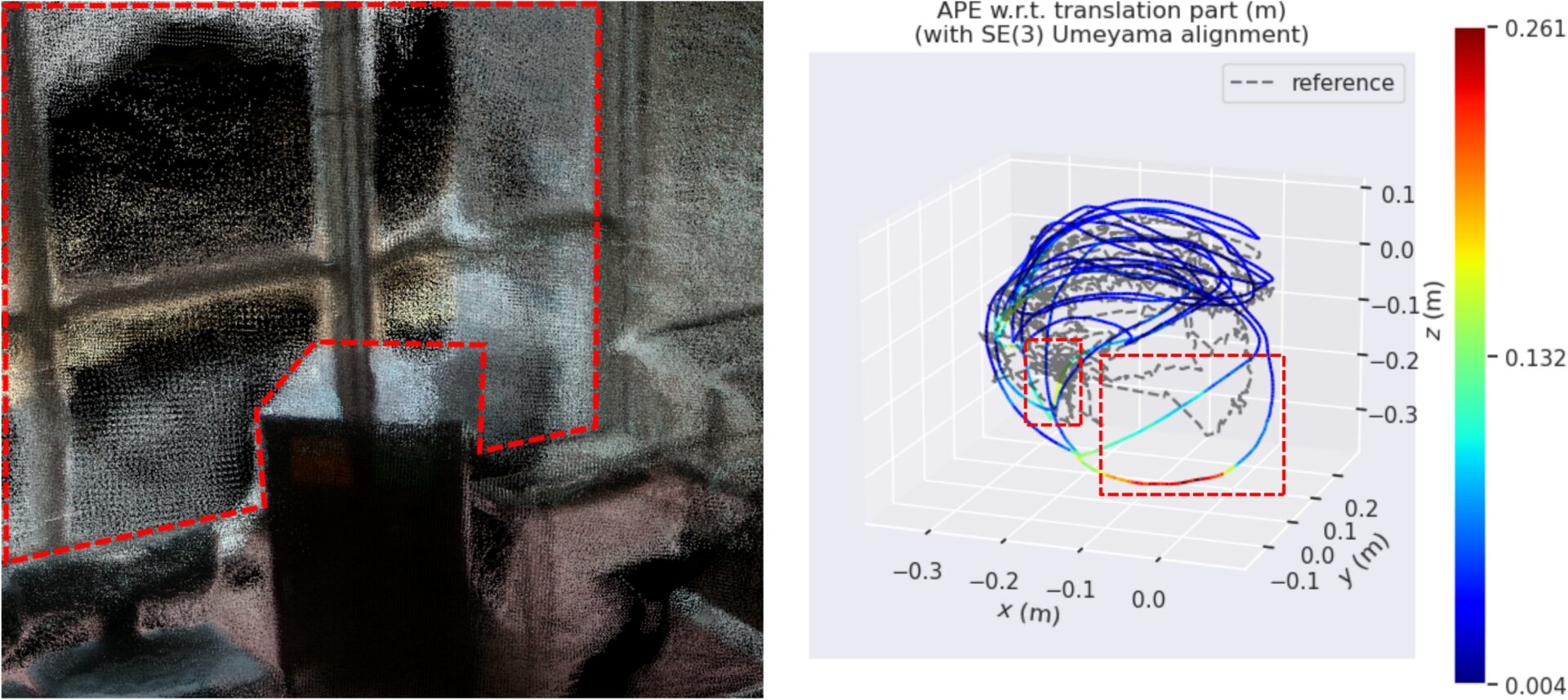
To improve the performance of existing RGB-D SLAMs in glass surface scenes, we propose a method based on ORB-SLAM2, named ORB-SLAM2-GSD, which performs well in such environments. Additionally, we introduce the first RGB-D dataset specifically designed to evaluate the performance of RGB-D SLAMs in glass surface scenes, named GS RGB-D. The dense reconstruction and camera trajectory estimation results of our proposed ORB-SLAM2-GSD are shown in the figure below.
ガラス表面シーンにおける既存のRGB-D SLAMの性能を向上させるために、ORB-SLAM2を基にした手法「ORB-SLAM2-GSD」を提案した。この手法は、ガラス表面が多い環境において優れた性能を発揮する。また、RGB-D SLAMのガラス表面シーンでの性能を評価するために設計された初のRGB-Dデータセット「GS RGB-D」を提案した。提案するORB-SLAM2-GSDによる点群地図作成およびカメラ軌跡推定の結果は、以下の図に示されている。
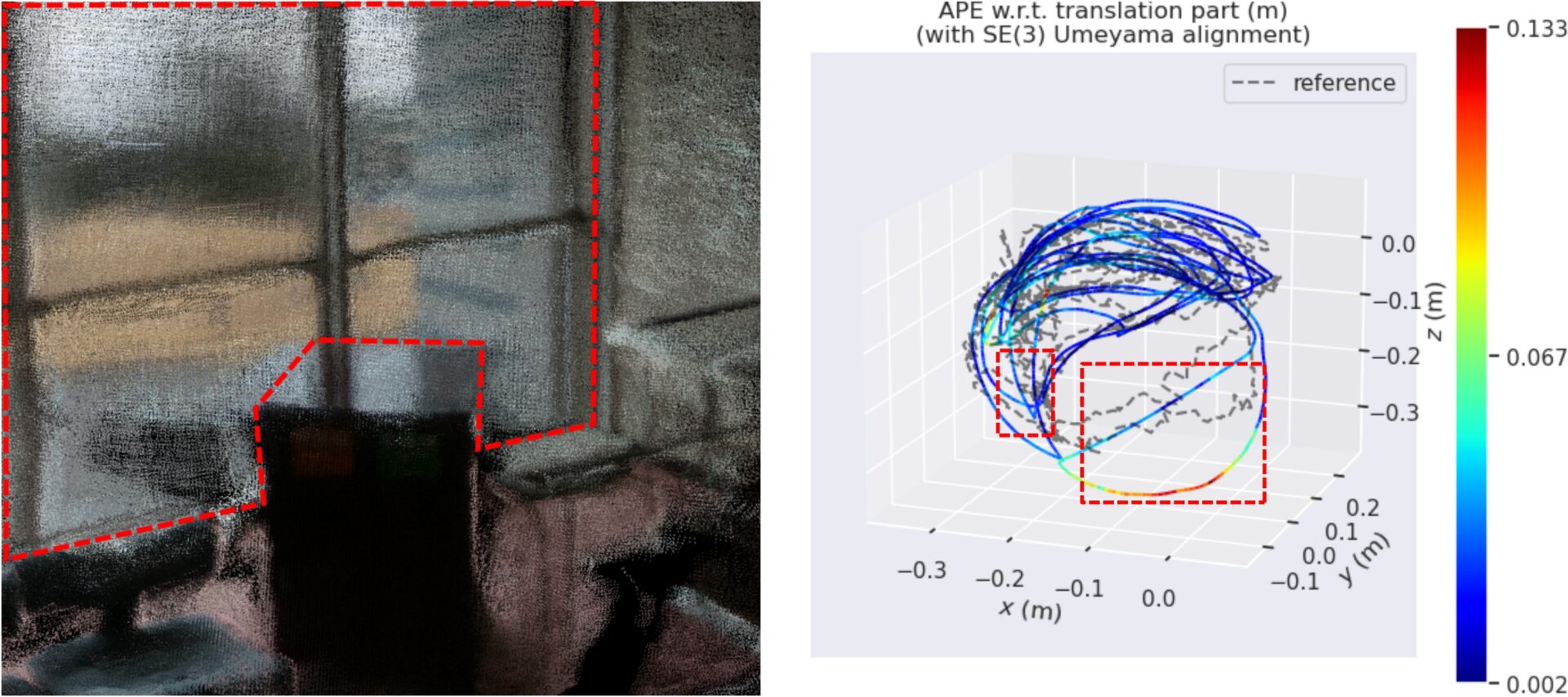
Publication
- Zeyuan Chen, Ziquan Wang, Qiang Gao, Masahiko Mikawa and Makoto Fujisawa, “Dense Reconstruction and Localization in Scenes with Glass Surfaces Based on ORB-SLAM2”, ICPR, 2024. DOI: 10.1007/978-3-031-78113-1_26.