In recent years, the shortage of human resources in the animation and video production industry has become a significant issue, leading to a demand for efficient video generation methods. Deep learning-based video generation techniques are seen as a potential solution, with research focusing on generating natural phenomena videos from single images. Progress has been made in developing video generation networks that incorporate external forces, such as wind, into the models. However, there is still a need for further advancements in creating networks that can more accurately and effectively respond to the creator’s intent and handle complex natural phenomena.
In this research, we constructed a video generation network that uses a single image of a flame and wind direction information as input to generate natural flame videos considering the external force of wind. This network was trained through a two-stage process based on VQ-VAE (Vector Quantized-Variational AutoEncoder) and Transformer models. The network demonstrated the ability to capture the relationship between flame motion and wind. The videos generated by this network accurately depicted changes in flame behavior due to wind direction, showcasing the high accuracy of the video generation. Furthermore, the Fréchet Video Distance (FVD) index achieved by our network was comparable to those reported in similar studies, indicating competitive performance.
近年、アニメーションや映像制作業界における人材不足が深刻な問題となっており、効率的な動画生成手法の需要が高まっている。深層学習を基盤とした動画生成技術は、その解決策の一つとして注目されており、特に単一の画像から自然現象の動画を生成する研究が進められている。風などの外力をモデルに組み込んだ動画生成ネットワークの開発も進展しているが、制作者の意図により的確に応じ、複雑な自然現象に対しても効果的に対応できるネットワークのさらなる進化が求められている。
本研究では、炎の単一画像と風向きの情報を入力として、風という外力を考慮した自然な炎の動画を生成するネットワークを構築した。このネットワークは、VQ-VAE(Vector Quantized-Variational AutoEncoder)とTransformerモデルに基づく二段階の訓練プロセスを通じて訓練された。ネットワークは炎の動きと風の関係性を捉え、風向きの変化に応じた炎の挙動を正確に描写する動画を生成する能力を示した。また、生成された動画の品質はFVD(Fréchet Video Distance)指標を用いて評価され、他の類似研究と比較しても遜色のない競争力のある性能を達成したことが確認された。
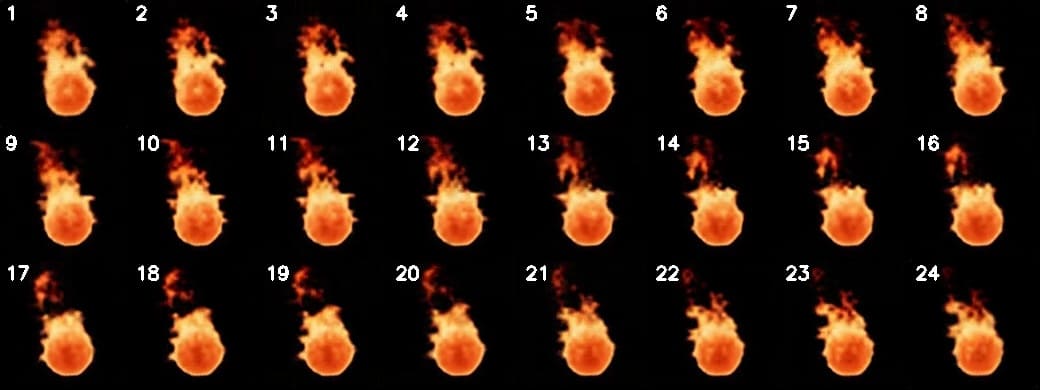
Publication
- Izuho Takahashi, Zeyuan Chen, Masahiko Mikawa and Makoto Fujisawa, “Video Generation with Single Flame Image and Wind Direction Information”, SCIS&ISIS, 2024.