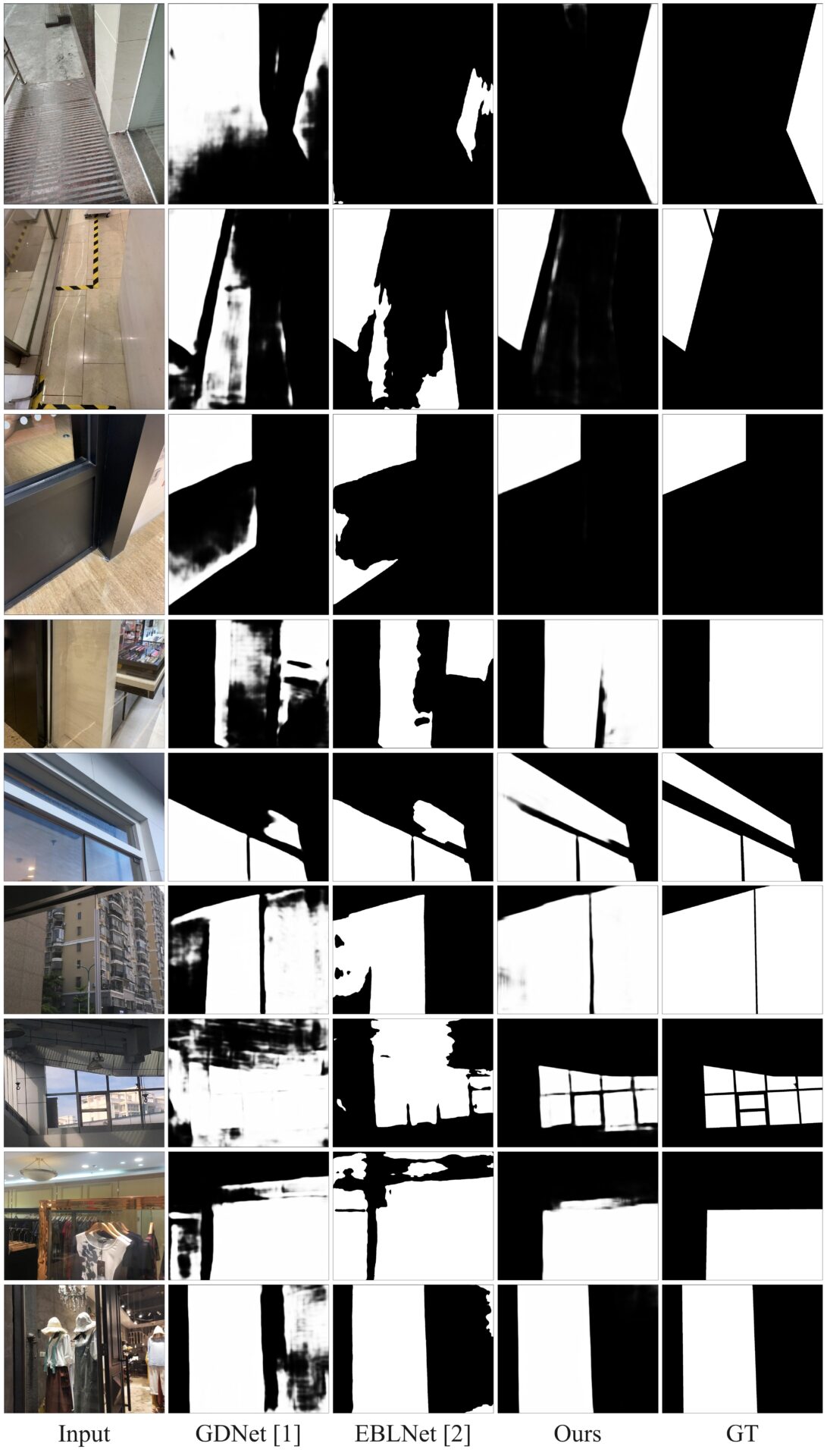
Glass surfaces, such as windows, are ubiquitous in our daily life. However, conventional object detection methods in the field of computer vision perform poorly in detecting them due to their transparent nature and varying shapes. In this paper, we propose a glass surface detection network named CGSDNet, which employs ConvNeXt backbone network and a novel Cascade Atrous Pooling (CAP) module to extract multi-scale contextual features (e.g., the difference between objects located inside and outside the glass, the reflection, texture and obstructed situation of the glass). Additionally, we present a lightweight Holistic Boundary Detection (HBD) module to capture boundary features from the contextual features. Finally, we propose a Cascaded Architecture to fuse the contextual features with the boundary features, generating dense large-field contextual features with enhanced boundaries, which are utilized for robust glass surface detection. Extensive experiments on benchmark datasets demonstrate that our proposed method outperforms state-of-theart (SOTA) methods from relevant fields.
ガラス表面、例えば窓などは、私たちの日常生活に広く存在している。しかし、コンピュータビジョンの分野における従来の物体検出方法は、透明性や形状の多様性のために、ガラス表面を検出する際に性能が低いである。本研究では、我々は CGSDNet というガラス表面検出ネットワークを提案する。このネットワークは、ConvNeXt バックボーンネットワークと新しい Cascade Atrous Pooling(CAP)モジュールを使用して、マルチスケールのコンテクスト特徴(例:ガラスの内側と外側にあるオブジェクトの違い、ガラスの反射、テクスチャ、遮られた状態など)を抽出する。さらに、軽量な Holistic Boundary Detection(HBD)モジュールを提案し、コンテクスト特徴から境界特徴をキャプチャする。最後に、コンテクスト特徴と境界特徴を融合するためのカスケードアーキテクチャを提案し、境界による強化された高密度の大規模コンテクスト特徴を生成する。これらの特徴は、ロバストのガラス表面検出に使用される。ベンチマークデータセットでの広範な実験により、我々の提案手法が関連分野の最先端の手法を上回ることが示されている。
Publication
- Zeyuan Chen, Masahiko Mikawa and Makoto Fujisawa, “CGSDNet: Cascade Network with ConvNeXt as Backbone for Glass Surface Detection”, IEEE ICAICA, 2023.